Language AI originally knew whether my answer was correct!New research on Berkeley and other universities
Author:Quantum Time:2022.07.15
Wanbo from the quantity of the Temple of the Temple | Public Account QBITAI
Language AI, with human self -examination ability:
Recently, a study from an academic team from the University of California Berkeley and Hopkins University showed that:
Not only can it judge whether its answer is correct or not, but after training, it can also predict the probability of knowing the answer to a question.
Once the research results were released, it caused heated discussion. Someone's first reaction was panic:
Some people think that this result is positive for neural network research:
Language AI has self -examination ability
The research team believes that if the language AI model is to be evaluated, there must be a premise:
When language AI answers questions, you will calibrate your answer.
The calibration here is the correct probability of a language AI prediction to an answer, whether it is consistent with the actual probability.
Only such a language AI can use this calibration ability to evaluate whether the answer you output is correct.
So the first question is, can language AI calibrate your answer?
To prove this, the research team has prepared 5 choices for AI:
The answer option is given in the form of A, B, and C.
If the accuracy rate of the AI model answer exceeds the chance of chance, then the answer given by the AI model is calibrated.
The result of the test is that the answer given by the language AI obviously exceeds the accidental probability of any option.
In other words, language AI models can calibrate their answers well.
However, the research team found that the calibration ability of language AI was based on the premise of clear option answers.
If you add an uncertain option to "above" in the option, it will damage the calibration ability of the language AI.
In other words, in the choice questions in a specific format, the language AI model can be calibrated well on the answer.
After clarifying this premise, the next question is to verify that the language AI model can determine whether its answer is correct.
In this round of tests, in order to make the AI model prediction closer to your effective decision -making boundary.
The research team still chooses the question of the previous round of tests and the answer sample of the language AI model.
At the same time, let the AI model choose the true and false of their own answers, and then analyze whether the AI model is made effective calibration for this "true" or "fake" answer.
Question settings, such as below:
After 20 authenticity tests, the research team found that the language AI model's evaluation of the answers or "true" or "fake" of the language AI model has been obviously calibrated.
In other words, if you ask several questions to the AI model within a scope, then the AI model has a reasonable evaluation of the answers to these questions, which is reasonable and calibrated.
This also proves that the language AI model can indeed determine whether it is correct for a question.
Finally, the research team raised a more difficult question for the language AI model: after training, the AI model can predict whether they know any answer to any given question.
In this session, the research group introduced a data P (IK) (I know the probability of this answer) and select one in the following two training methods for training:
Value Head (value orientation): P (IK) is trained into an additional value orientation, and then added to the number of models (independent to the number of language modeling, the advantage of this method is that the research team can be easy to be easy Detecting the general labeling position of P (IK). Natural Language: This method is relatively simple to request the AI model to answer "what is the probability of this answer" literally, and at the same time output a percentage data answer.
In the early days of training, the research team is more inclined to natural language training, but the results are not significant, and from this to the value -oriented method, the research team also stated that the training of AI models will return to natural language methods.
After training, the research team found that the language AI model can well predict P (IK), and in different types of problems, this predictive ability is partially versatile.
However, the research team also found that in certain types of problems, such as arithmetic problems, language AI models have some difficulties during OOD calibration.
For this academic achievement, the research team stated that the future direction is to promote these achievements and the language AI model without imitating human text, self -learning and factual reasoning.
about the author
The thesis communication author, Dr. Jared Kaplan, is a theoretical physicist and a machine learning expert. He is currently a assistant professor of Hopkins University. The main research field, machine learning research, including the enabilities of neural models and GPT- 3 Language model.
Co -communication author Saurav Kadavath, researcher of Anthropic, now studying for a master's degree in EECS, EECS, the University of California. The main research field is machine learning, large -scale language learning, etc.
Reference link:
https://arxiv.org/abs/2207.05221
- END -
Beijing high -quality construction of "dual hub" international consumer bridgehead
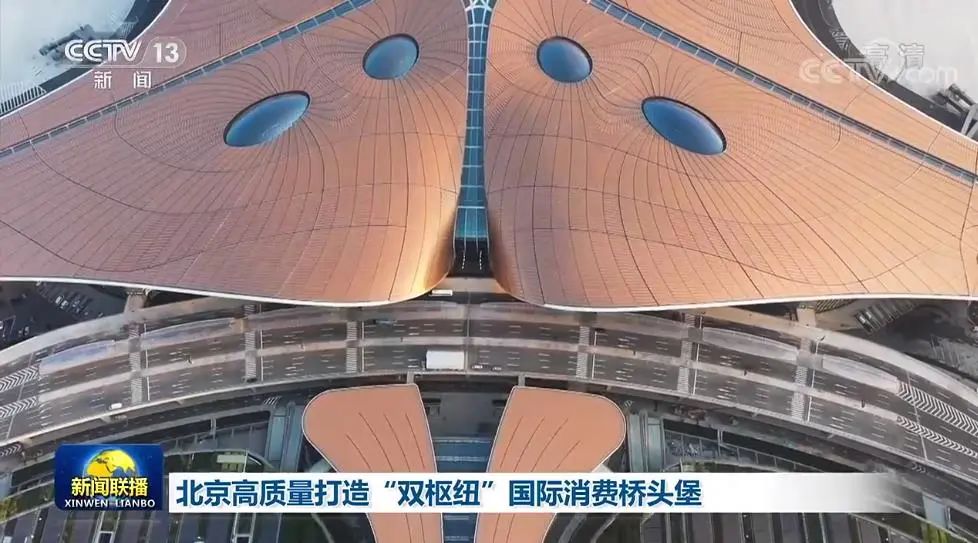
Beijing actively cultivates and constructs international consumer cities, gives...
Hanying Street Sunshine Community holds family education to enter the community activities
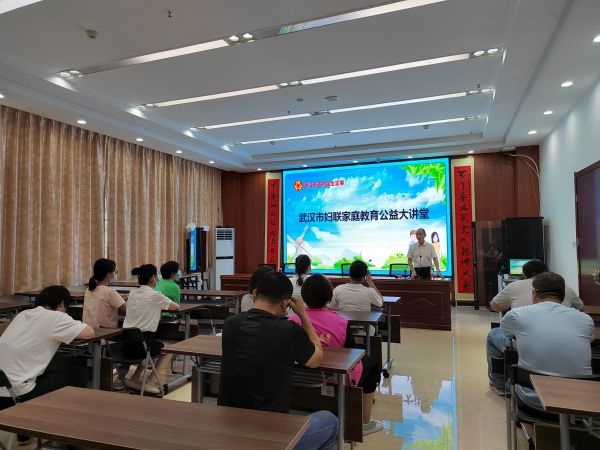
The Yangtze River Daily Da Wuhan Client July 10 (Correspondent Li Jing) Parents of...