Machine translation is to make up for the gap between human -machine translation
Author:Catti center Time:2022.07.31
To what extent should we believe that the machine is actually learning to understand the semantics slowly, or is the accuracy of machine translation approaching the level of human beings? To answer this question, let us look more carefully to see the facts based on these statements. First of all, we should figure out how these companies measure the translation quality of a machine or a person. The quality of the translation is not so simple. A given text can have many correct translation methods. Of course, there are more wrong translation methods. Because there is no unique correct answer to a given text, it is difficult to design a method that can automatically evaluate the accuracy of the system translation.
Google claims that its new method launched in 2016 to make up for the gap between people and machine translation. Several other large technology companies caught up and created their own online machine translation procedures, which are also based on the architecture of the encoder-decoder. These companies and the technology media reported on them are enthusiastically promoting these translation services. "MIT Science and Technology Review" magazine reported: "This new service of Google can almost translate language like humans." Microsoft said at a company promotion conference that the level of Chinese translation services for English news has been comparable to humans. IBM claims: "Watson can speak 9 languages fluently, and this number is still increasing." Facebook is responsible for language translation executives frankly: "We believe that neural networks are learning the potential semantics of language." Professional translation company Deepl's CEO bragged: "Our machine translation neural network has developed an amazing understanding."
In general, these statements are promoted by the competition of sales in sales to a certain extent by technology companies, and language translation is one of the main services with great profitable potential. Although websites such as Google Translation will provide free translation services for a small number of texts, if a company wants to translate a large number of documents or provide translation for customers on its own website, you need to use charging machine translation services. All these services are all services. All these services are all services. All these services are all services. All these services are all services. Support it from the same encoder-decoder architecture.
With the introduction of deep learning, the level of machine translation has been greatly improved. So can it prove that the machine translation is now close to the level of human beings? In my opinion, this statement is unreasonable from several aspects. First, the average number of scores will be misleading. For example, for machine translation, although the translation of most sentences is rated as "good", many sentences are rated as "bad", then its average level is "good". However You may want a translation system that is always well performed well, never wrong, and more reliable.
Secondly, these translation systems are close to the level of human beings or are equivalent to human levels based on its assessment of a single sentence translation level, rather than the translation of longer articles. In an article, sentences are usually interdependent in important ways, and these may be ignored in the process of translation of a single sentence. I haven't seen any formal research on the assessment of the long text of the machine translation. Generally speaking, the quality of the long text of the machine translation will be a little worse. For example, for Google Translation, the entire paragraph is given instead of a single sentence. At the same time, its translation quality will decrease significantly.
Finally, these assessment sentences are extracted from news reports and Wikipedia pages. These pages are usually carefully written to avoid ambiguous languages or practices. Such a language may bring serious problems to the machine translation system, but it cannot be avoided in the real world.
Lost in translation
Remember the story of "Restaurant Encounter" mentioned at the beginning of the previous chapter? I did not design this story to test the translation system, but this story does clearly clarify the challenges facing the machine translation system -spoken language, Xi, and ambiguous language.
I translated "Restaurant Encounter" in Google translated from English into three target languages: French, Italian and Chinese. I sent the result of not using the original text to those friends who understand English and target language at the same time, and ask them to translate the translation result of Google's translation back to English in order to understand a person who is proficient in the corresponding target language. What is obtained in the translated text. Here, for the reader's reading fun, I listed some translation results below.
Original story:
A man went into a restaurant andordered a hamburger, cooked rare. When it arrived, it was burned to a crisp. Thewaitress stopped by the man 'stable. “Is burger okay? “she asked. “Oh, it's justgreat,” the man said, pushing back his chair and storming out of the restaurantWithout paying. The waitress yelled after him, “Hey, what about the bill?” She shruggedher shoulders, muttering under her breath, “Why is he so bent out of shape?“( A man walked into a restaurant and ordered a burger bag for two points. When the burger bag was on the table, it was baked. The female waiter stopped at the man's table and asked: " Is the burger bag okay? "" Oh, it's so good! "The man said, pushing the chair to the back, and rushed out of the restaurant without paying. Do you? "She shrugged and whispered:" Why is he so angry? ") The French version provided by Google translated, and then the version of it manually translated it into English:
English version
A man entered a restaurant andordered a hamburger, cooked infrequent. When he arrived, he got burned at acrunchy. The waitress stopped walking in front of the man' stable. “Is the hamburgerdoing well? “She asked. “Oh, it' terrific ,” said the man while putting his chairback and while going out of the restaurant without paying. The waitress shoutedafter him, “say, what about he proposed legislation? “She shrugged her shoulders,mumbling in her breath,“Why is he so distorted ? "(A man walked into a restaurant and ordered a burger bag, which was rare. When he arrived, he was burnt and made a crunching noise. The female waiter stopped at this person's table. "Is the burger bag doing well?" She asked. "Oh, it's great!" The man walked out of the restaurant while putting the chair back. What about the regulations? "She shrugged and murmured to herself:" Why is he so distorted? ")
Reading these translations is like listening to a talented but frequent error pianist to play a melody that we are familiar with. This melody is generally recognizable, but it is fragmented and uncomfortable. This song is short in short short. When the outbreak, it was very beautiful, but it was always interrupted by the harsh error notes. Google translation sometimes chooses the meaning of errors on some versatile words, such as translating "rare" (semi -familiar) and "bill" into "uncommon" and "proposed regulations". It happened mainly because the program ignored the context of these words. "Burnedto A CRISP" and "Bent Out of Shape" are strange. This program seems to be unable to find the corresponding Xi in the target language, or figure out the Xi. The actual meaning.
Although these translations have expressed the outline of the story, some subtle and important parts are lost in all translation versions, including expressing men's anger as "rushing out of the restaurant", and the dissatisfaction of the female waiter's dissatisfaction. Expression is "muttering", let alone the correct grammar occasionally lost during the translation process.
I am not interested in picking Google's translation problems. I also tried many other online translation services and got similar results. This is not surprising, because these systems use almost the same encoder -decoder architecture. In addition, I want to emphasize one thing: the translation results I get represents the stage level of these translation systems at a certain time. Their translation ability has been continuously improved. The above translation errors may be It was repaired. However, I still think that the level of machine translation to truly reach the level of human translator has a long way to go, except in some specific segments. For machine translation, the main obstacle is: Like the problem of the voice recognition system, the machine translation system does not really "understand" the text they are dealing with when performing tasks. In translation and voice recognition, there have always been such problems: To what extent the machine needs to have this understanding ability to reach the level of human beings? Hou Shida believes: "Translation is much more complicated than checking dictionary and re -arrangement ... To do a good job of translation, machines need to have a psychological model to the world they discuss." Such a psychological model: When a person leaves the restaurant without paying, the waiter is more likely to yell at him to pay the bill, instead of saying some proposal regulations. Hou Shida's view was responded in an article by artificial intelligence researchers Ernest Davis and Macos in 2015: "Machine translation usually involves some ambiguity issues, only to reach right. The true understanding of the text can complete this task with real world knowledge. "A encoder-decoder network can simply get the necessary psychological models and pairs through exposure to greater training sets and building more network layers Do you know the real world? Or do I need to pass some completely different methods? This is still an unreasonable issue, and it is also the theme of fierce debate in the artificial intelligence research group. Now, I just want to say that although the translation of neural machines is very effective and practical in many applications, but if there is no knowledge of human beings for post -editing, they are fundamentally unreliable. Therefore, we are more or less doubtful when using machine translation. For example, when I use Google to translate "take it with a grain of salt" (doubt about the results) translated from English to Chinese, and then translated back to English, it becomes "bring a Salt Bar" " (Bring a salt bar), it is really interesting.
Translate the image into a sentence
There is a crazy idea: In addition to translation between languages, can we train a neural network similar to the encoder-decoder architecture, so that they can learn to translate images into language? The idea is: first use a network to encode the image, and then use another network to "translate" it into a sentence describing the image content. After all, isn't it a translation method to create a title for an image? It's just that the source and target language in this case are a painting and a title, respectively.
It turns out that this idea is not so crazy. In 2015, two teams from Google and Stanford University published very similar papers on each other at the same computer visual conference. Here, I will describe a system called "Show and Tell" developed by Google team, because it is simpler in concept.
Figure 12-2 gives the working principle framework of this system Figure 22. It is similar to the encoder decoder system in Figure 12-1, but the input here is an image rather than a sentence. The image is input into a Convoral NET instead of the encoder network. The CONV Nets here is similar to the one I described in Chapter 04, but this CONV Nets does not output the classification of images. The activation value of the network decoding output a sentence. In order to encoding images, the R & D team uses a Conv NETS trained by image classification tasks that IMAGNET (I have described in Chapter 05). subtitle.
How does this system learn to generate suitable subtitles for images? Recall the principle of mutual translation between different languages: the training data consists of a pair of sentences. The sentence uses the source language for the first sentence in the sentence. the result of. In the same way, in the case of generating subtitles for images, each training sample consists of a image and a section of subtitles that matches it. These pictures are downloaded from public image repository such as Flickr, and their subtitles are generated by Google's Amazon Turkish robot hired by Google for this study. Because the subtitles can be very varied, each image is given a section of subtitles by 5 different people. Therefore, each image will appear 5 times in the training concentration, each time pairing with different subtitles. Figure 12-3 shows a training image sample and subtitles provided by Amazon Turkey robots. This "SHOW and Tell" decoder network trained about 80,000 "Image-Subtitles" samples. Figure 12-4 gives some subtitle samples generated on the test image. These test images are not concentrated on the system.
A machine can generate such accurate subtitles for images composed of pixels. This performance is too bright and shocking. This is the first time I see these results in the New York Times. The author of that article was John Mark Off [2], who wrote a cautious comment article, one of which was written like this: "The two groups of scientific research teams work independently, respectively, The artificial intelligence software that can identify and describe the content in the video and describe the content of photos and videos is higher than that at any time before, and sometimes it can even comparable to human understanding. "
However, some media are not so rigorous. A news website claims: "Google's artificial intelligence now adds subtitles to images is almost as good as humans." Other companies also quickly used similar automatic techniques to add subtitles to the image and issued a statement. Microsoft claims that "Microsoft researchers are in the forefront of the following technology research and development: automatic identification of objects in images, the situation of interpreting images, and writing an accurate explanation for it." Microsoft even created an online prototype system for their system called "CaptionBot". The website of CaptionBot claims: "I can understand the content of any photo, and I will strive to do it as well as humans."
Companies such as Google, Microsoft and Facebook have begun to discuss, to how much this technology can be applied to providing automatic image identification for people with vision disorders. There is a problem of polarization of the same performance as the translation of the machine as the translation of the machine. When it performs well, it looks almost amazing, as shown in Figure 12-4, but it can also be wrong from the theme to the complete nonsense. Figure 12-5 shows some examples of examples. Essence These wrong subtitles may make you laugh, but for a blind man who cannot see these photos, you cannot judge whether these subtitles are good or bad.
Although Microsoft said that its CaptionBot can "understand" any photo content, it is exactly the opposite: even if the subtitles they give are correct, these systems cannot understand the image from a human perspective. When I show Microsoft's CaptionBot at the beginning of Chapter 4, the output of the system is: a man holds a dog. That's right, except for the "men" part ... it is even more regrettable that this description missed the key, valuable points in the photo, and missed the experience of us, the experience of us, and the experience of us. How to express our emotions and knowledge about the world, that is, it missed the significance of this photo.
I am convinced that the capabilities of these systems will be improved as researchers apply more data and algorithms. However, I think that the content of the subtitle generation network still lacks a basic understanding of the content in the image, which is inevitable: just like a machine translation In the same performance, these systems are still unbelievable. They run well in some cases, but sometimes they are disappointing. In addition, even if these systems are mostly correct, they often cannot grasp the main points for a rich image.
Classify the sentence according to emotions, translate documents, and describe photos. Although the level of natural language processing systems on these tasks is far less than humans, it is still useful for the completion of many tasks in the real world. Therefore, for R & D personnel, This work has a lot of room for profit. However, the ultimate dream of natural language processing researchers is that the design can interact with users in real time to make smooth and flexible interaction with users, especially to talk to users and reply to their questions.
Source: Michelle Merana's new book "AI3.0"
Transfer from: human -machine and cognitive laboratory
- END -
Yuanhui Community: Reading Red Classic Inheritance Red Spirit
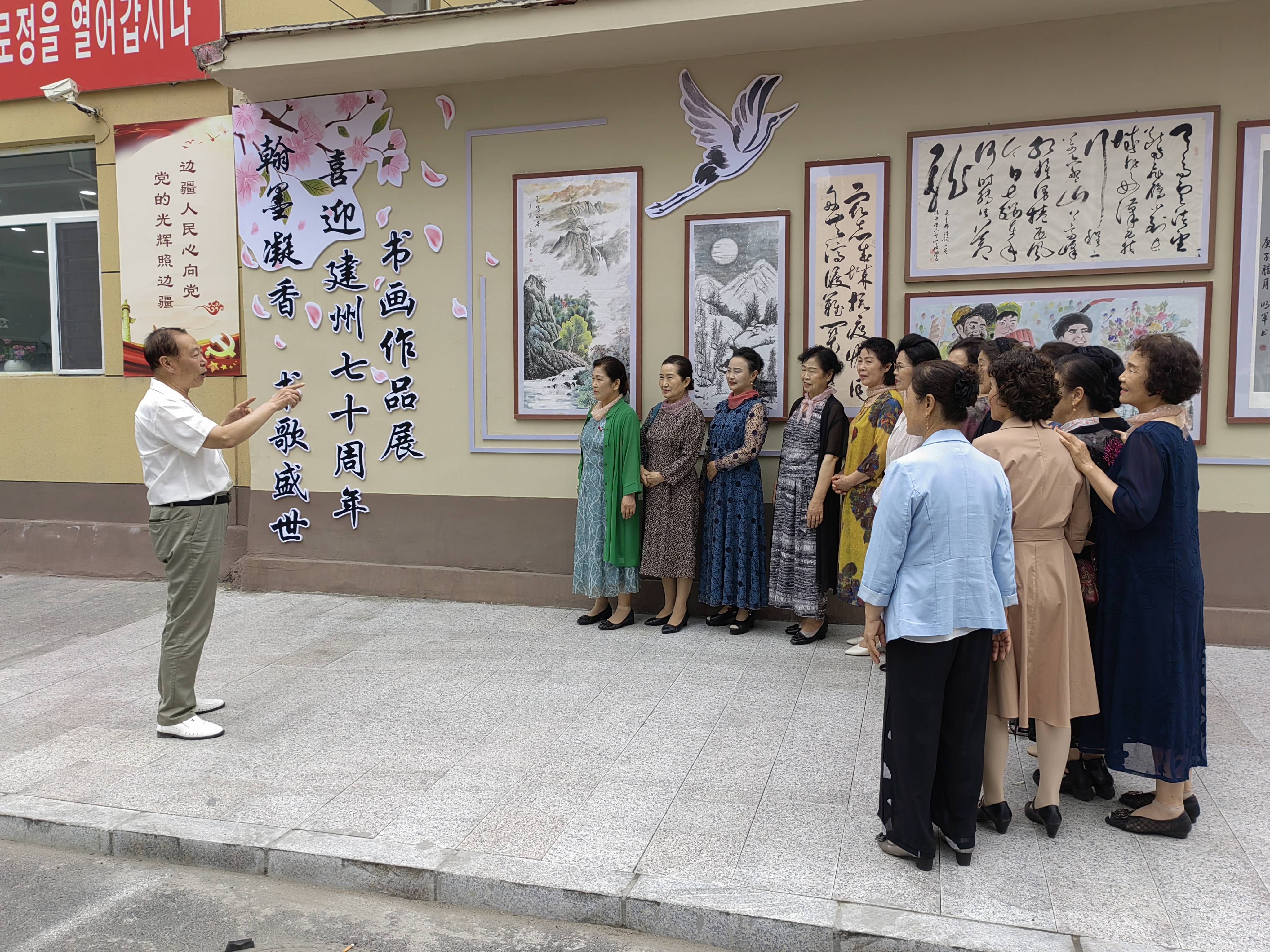
On July 12, the Party Committee of the Gaohui Community Party Committee of Park St...
Gongyi Municipal Meteorological Bureau issued high -temperature orange warning [level/severe]
Gongyi City Meteorological Observatory, June 08, 2022, at 08:56 at 08:56, a high -temperature orange warning signal: Today, the highest temperature of all towns and streets in Beishankou Town, Gongyi