Meta AI under Lecun's leadership, bet betrayal self -supervision
Author:Data School Thu Time:2022.07.23
Source: Heart of the machine
This article is about 1500 words, it is recommended to read for 5 minutes
Meta's MAE is based on a neural network architecture called Transformer.
Self -supervision learning is really a key step leading to AGI?
Meta's AI chief scientist, Jann Lecun, did not forget the long -term goals when talking about specific measures to be taken at this moment. In an interview, he said: We want to build smart machines like animals and humans.
In recent years, Meta has published a series of papers on AI System Self -Supervision (SSL). Lecun firmly believes that SSL is a necessary prerequisite for the AI system. It can help the AI system build a world model to obtain the ability of similar human beings, such as rationality, common sense, and the ability to migrate skills and knowledge from one environment to another.
Their new thesis shows a self -supervision system called a mask self -encoder (MAE). Although MAE is not a new idea, Meta has expanded this work to a new field.
Lecun said that by studying how to predict lost data, whether it is static images or video or audio sequences, the MAE system is building a world model. He said: If it can predict what is about to happen in the video, it must understand that the world is three -dimensional, some objects are lifeless, they will not move, other objects have life, it is difficult to predict, until predicts are predicted that there are some predictions, there are some predictions, there are predictions that there are some predictions. The complicated behavior of life. Once the AI system has a precise world model, it can use this model to plan.
Lecun said the essence of intelligence is to learn prediction. Although he did not claim that META's MAE system was close to GM artificial intelligence, he believed that this was an important step leading to general artificial intelligence.
But not everyone agrees with Meta researchers on the correct path to GM artificial intelligence. Yoshua Bengio sometimes debates friendly with Lecun on the major ideas in the AI field. In an email to IEEE SPECTRUM, Bengio explained some of their differences and similarities on the goals.
Bengio wrote: I really don't think our current method (whether it is self -supervision) is enough to bridge the gap between manual and human intelligence levels. He said that the field needs to achieve qualitative progress in order to truly promote technology to move closer to human -scale artificial intelligence.
For Lecun's reasoning ability to inference the world is the core element of intelligence, Bengio agrees, but his team did not focus on the model that can predict, but instead put knowledge in the form of natural language to present knowledge On the model. He pointed out that such a model will allow us to combine these knowledge fragments to solve new problems, carry out anti -fact simulation, or study possible future. Bengio's team has developed a new neural network framework, which is more modular than the framework favored by LECUN dedicated to end -to -end learning.
TransFormer of the fire
Meta's MAE is based on a neural network architecture called Transformer. This architecture initially became popular in the field of natural language processing, and then expanded to multiple fields such as computer vision.
Of course, Meta is not the first team to successfully use Transformer in visual tasks. Researcher Ross Girshick of Meta AI said that Google's research on vision transformr (VIT) inspired the META team, and the use of VIT architecture to help (we) eliminates some obstacles encountered during the test.
Girshick is one of the authors of the first MAE system papers in Meta. One of this paper is He Kaiming. They discuss a very simple method: cover the random block of the input image and rebuild the lost pixels.
The training of this model is similar to Bert and some other transformer -based language models. Researchers will show them a huge text database, but some words are missing or covered. The model needs to predict the missing words by itself, and then the covered words will be revealed, so that the model can check their work and update their parameters. This process will be repeated. Girshick explained that in order to do similar things visually, the research team decomposed the image into patch, and then covered some patches and asked the MAE system to predict the lack of image.
One of the breakthroughs of the team is to realize that most of the images will obtain the best results, which is a key difference between language transformer, and the latter may only cover 15% of words. Language is an extremely dense and efficient communication system. Each symbol contains many meanings. Girshick said, but the images -these signals from the natural world -are not built to eliminate redundant. That's why we can compress the content well when creating a JPG image. Researchers of Meta AI test how many images need to be masked for the best results.
Girshick explained that by covering more than 75% of Patch in the image, they eliminate redundancy in the image, otherwise the task will be too trivial and not suitable for training. Their MAE system consisting of two parts first uses an encoder to learn the relationship between pixels by training datasets, and then a decoder can make a maximum effort to reconstruct the original image from the masking image. After the training scheme is completed, the encoder can also be fine -tuned for visual tasks such as classification and target detection.
Girshick said that the end of our excitement was that we saw the result of this model in the downstream task. When using the encoder to complete the tasks such as target recognition, the benefits we see are very considerable. He pointed out that continuing to increase the model can get better performance, which is a potential direction for future models, because SSL has the potential to use a large amount of data without manual annotation.
Learning a large number of unbiased data sets may be the strategy of improving SSL results in META, but it is also an increasingly controversial method. Artificial intelligence ethics researchers such as Timnit Gebru have called on everyone to pay attention to unprecedented prejudice in the unreasonable data sets of large language models, which sometimes lead to catastrophic results.
Self -supervision learning of video and audio
In the video MAE system, the mask covers 95%of each video frame, because the similarity between the frames means that the video signal has more redundancy than static images. Meta researcher Christoph Feichtenhofer said that as far as video is concerned, a major advantage of the MAE method is that videos usually require a lot of calculations, and MAE reduces up to 95% of the calculation cost by blocking 95% of each frame.
The video clips used in these experiments are only a few seconds, but Feichtenhofer said that training artificial intelligence systems with longer video training is a very active research topic. Imagine that you have a virtual assistant. He has a video of your home that can tell you where you put the key a hour ago.
More directly, we can imagine that image and video systems are useful for the classification tasks required for content review on Facebook and Instagram. Feichtenhofer said that Integrity is a possible application. We are communicating with the product team, but this is this is this, but this is this is that this is this, but this is this. Very new, we have no specific project yet.
For the audio MAE work, the Meta AI team said they will soon publish the research results on Arxiv. They discovered a clever method to apply covering technology. They convert sound files into sound spectrum, that is, the visual characteristics of the signal mid -frequency spectrum, and then cover some images to train. The rebuilding audio is impressive, although the model can only process a few seconds of fragments.
Researcher Bernie Huang of the audio system said that the potential applications of this research include classification tasks. The audio lost by filling the data packet to the DROP to assist IP -based voice transmission (VOIP), or find more effective compressed audio files method.
Meta has been conducting open source AI research. For example, these MAE models also provide a large -scale language model for the artificial intelligence community. However, critics pointed out that despite being so open in research, Meta has not yet opened its core business algorithm for everyone to study, that is, those algorithms that control news push, recommendations and advertising implantation.
Original link:
https://spectrum.ieee.org/unsupervised-rearning-Meta
Edit: Yu Tengkai
- END -
People's Daily | Kindergarten at the door of the Village Wafa Home
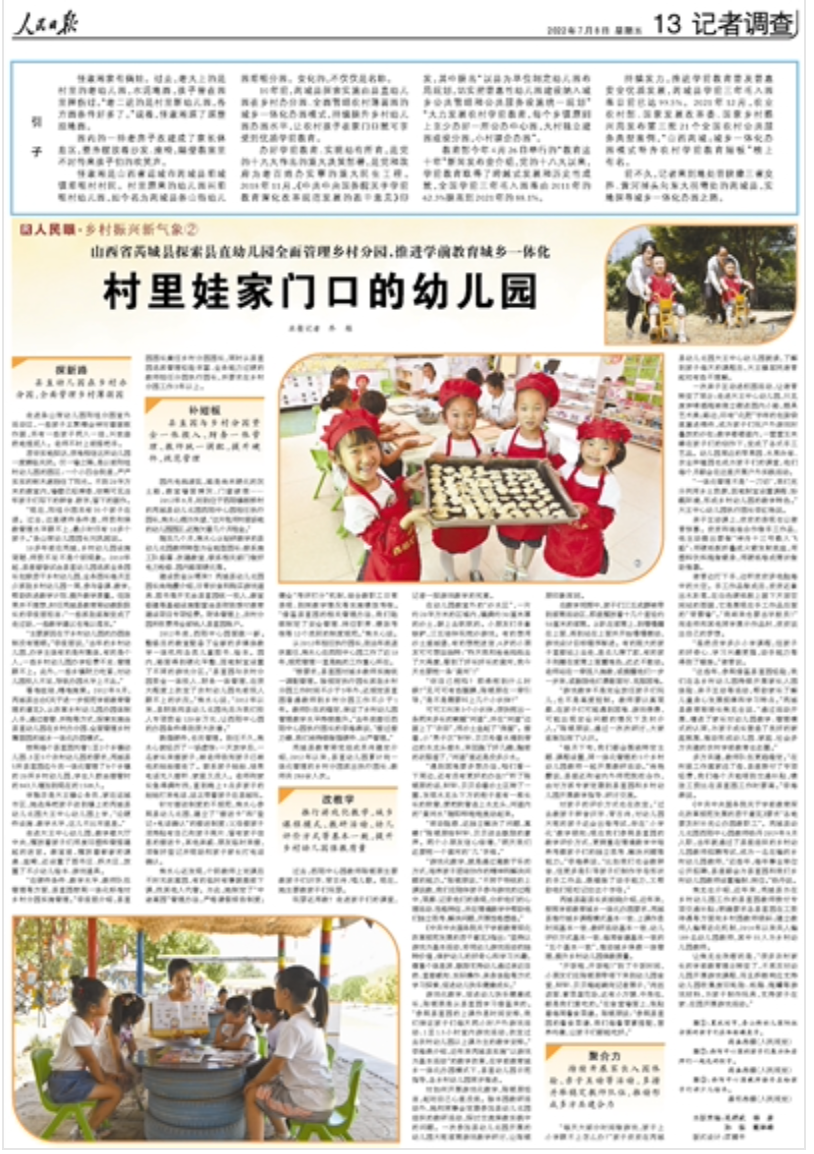
People's Daily (July 08, 2022, Edition 13)Ruicheng County, Shanxi Province explore...
Western Union Langalian Autonomous County Meteorological Observatory to remove lightning yellow warn
According to the latest meteorological data analysis, the West League County Meteorological Station was lifted at 23:45 on June 9, 2022 to release a lightning yellow warning signal issued at 17:45 on