[Harvard University-Cell] Analysis of pan-intensive tissue genome through multi-modal deep learning
Author:Data School Thu Time:2022.08.13
Source: Specialty
This article is introduced in the paper. It is recommended to read for 5 minutes
We use multi -modal deep learning joint examinations from 14 cancer types of pathological and molecular cutting data.
The rapid appearance of computing pathology has proven that there is an objective prognostic model from the development of historical images. However, most prognostic models are either based on histology or only based on genomics. It has not solved how to integrate these data sources to develop combined image group prognostic models. In addition, it is also meaningful to control this prognosis from these models that can be explained and molecular descriptive. We use multi -modal deep learning joint examinations from 14 cancer types of pathological and molecular cutting data. Our weak supervision and multi -mode deep learning algorithm can integrate these heterogeneous models to predict the results, and find the prognostic features related to adverse and favorable results. In a interactive open database, we analyzed all the morphology and molecular correlations of the prognosis of the 14 cancer types in the disease and patient level to allow further exploration, biomarker discovery and characteristic assessment.
Cancer is defined by the iconic tissue pathology, genome, and transcription groups in tumor and tissue micro -environment. These heterogeneousness helps to treat relief rates and variants of patient results (Marusyk et al., 2012) Essence At present, many cancer types of clinical models include manual assessment of tissue pathological characteristics such as tumor invasion, inter -degeneration, necrosis, and wire division, and then use them as classification and installment standards to divide patients into different risk groups in order to make treatment decisions for treatment decisions Essence For example, in tumors, lymph nodes, and metastases (TNM) staging systems, primary tumors are divided into different stages according to the severity of the tumor (such as size, growth, and heterogeneity). , Radiotherapy dose and other treatment decisions (amin et al., 2017). However, the subjective interpretation of the characteristics of tissue pathology has a large variability between the observer and the observers, and patients at the same grade or stage still have significant variability in the results. Although many tissue pathological students logo for diagnostic tasks has been established, most of them are based on the shape and location of tumor cells. How to affect the fine understanding of patients' risks (Marusyk et al., 2012; Chang et al., 2013; Heindl et al., 2015; Kather et al., 2018; TARANTINO et al., 2021).
The recent progress of deep learning in computer pathology has enabled the full -slicing image (WSIS) to be used for automatic cancer diagnosis and tumor microenvironment. Using weak supervision and learning, SLIDE clinical annotations can be used to guide deep learning algorithms to summarize conventional diagnostic tasks, such as cancer testing, classification, and subtype (Campanella et al., 2019; Lu et al., 2021). Although this algorithm can achieve the same performance as human experts on a narrow sense, quantification of the new prognosis morphological characteristics is limited, because training with subjective human beings may not be able to extract attributes that have not been recognized so far. Attributes can be used to improve the prognosis of patients (Echle et al., 2021). In order to capture the more objective and prognostic morphological characteristics that have not been extracted in the conventional clinical work process. Recently, based on deep learning methods, it is recommended to use results -based labels for supervision. For example 2019; Courtiol et al., 2019; Kather et al., 2019A, 2019b; Kulkarni et al., 2020; wuClzyn et al., 2020, 2021). In fact, recent work shows that the use of deep learning to automatically discover new and prognostic morphology The biomarkers have great potential (Beck et al., 2011; Echle et al., 2021; Diao et al., 2021 To.
https://www.cell.com/cancer-cell/pdfextended/s1535-6108 (2)00317-8
- END -
Latest notice of the Office of Rizhao Epidemic Prevention and Control Headquarters
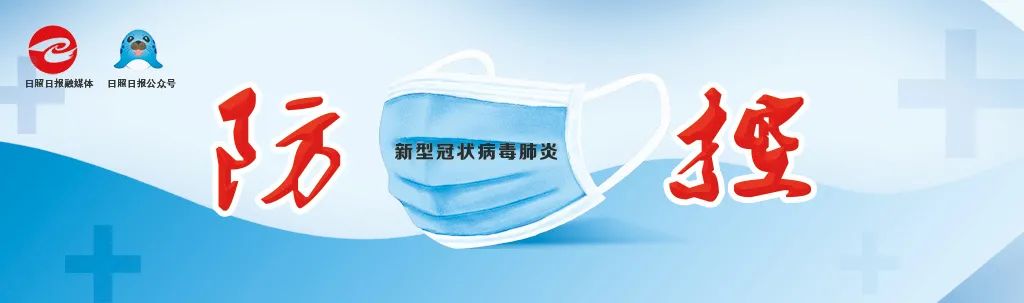
Notice on further strengthening the prevention and control of corporate epidemic c...
up to date!Health Tips for Health Centers for Disease Prevention and Control Center in Shanxi Province
At present, the situation of the epidemic situation in my country's new crown pneumonia is better, but the epidemic has not completely blocked. In order to continue to adhere to the general strategy o...