Thesis recommendation: Use a twin network with a mask for self -supervision and learning
Author:Data School Thu Time:2022.09.18
Source: Deephub IMBA
This article is about 1100 words, it is recommended to read for 9 minutes
This article introduces how to supervise the network with mask.
Recently self -supervision learning has been valued. Yesterday I discovered this job through LinkedIn, and I think it is very interesting. The MAE of the great god of Kaiming creates a new direction for VIT and self -supervision. This article will introduce Masked Siamese Networks (MSN), which is another self -supervision learning framework for learning image representation. MSN matchs the representation of an image view containing a random mask to match the representative of the original unmembling image.
Consider a large unsigned image set d = (x_i) and a small comment image set s = (x_si, y_i), where is Len (D) && Len (s). Here, the image in s may overlap in the image in the data set D. Our training goal is to learn vision representation by pre -training D, and then use S to indicate the transfer/fine -tuning to the supervision task.
Masked size networks
If you are more familiar with VIT, the content to be discussed below should be familiar. We convert each view into a series of non -overlapping NXN blocks "Patchs". The author then introduced the image through some random mask and obtained an enhancement of a image. In the figure above, you can see two strategies. Whether we use that strategy, we get the target sequence after the part (Patch) X+_i and its corresponding mask sequence x_i, m, of which the latter will be significantly shorter than the target than the target than the target Essence
The goal of the encoder VIT is to learn the representation of mask. Finally, a sequence is expressed by [CLS] token.
The author introduces a matrix Q, which is composed of K (K & 1) a learning prototypes, and the dimension of each prototype is D. First of all, we have the representation of the PatchFied Mask and the PatchFied only, Z_i, M and Z_i. Then use the L2 normalization of this representation, the corresponding prediction (P) is calculated by measuring the string similarity of the prototype matrix Q. TAU represents a temperature parameter, between (0,1). Note that the author uses a larger temperature value when calculating the target prediction, which guides the network to generate self -confidence low -entropy anchor forecasts.
Finally, the target function is
The first item represents the standard cross-entropy loss (H), and the second is the MIN-MAX regularization device. It seeks to maximize all mask sequences (P_i, M), and the average predicted entropy of P_HAT.
You need to pay attention to 3 questions here:
1. Calculate the gradient for the anchor prediction P_i and M. 2. In the standard comparison learning, the two views are clearly encouraged. MSN can do this by encouraging two views and learning prototypes, which can be regarded as a kind of cluster quality. The two views should fall into the same point in the embedded space. In addition, the number of prototypes can be learned in the super parameter. The author uses 1024 (matching the size of the batch), and the dimension D is set to 256.3 and MAE. However, MAE attempts to rebuild the image from its masked view, and MSN directly attempts to maximize the similarity of the two views.
result
MSN is better than MAE and other models on the linear assessment on ImageNet-KK. And MSN and MAE are interesting because they both introduce masks. This may be a visible discovery of the future work in this field. The author also found that the Focal Mask strategy will reduce performance, and Random Mask usually improves performance. But at the same time, the two will have significant improvements. do you remember? MAE only uses random cover.
Finally, when the model is increased, the author finds that the increase of the mask (discarding more pieces) can help improve the performance of the small sample.
I hope you think this article is helpful and/or interesting to your learning. The thesis address is here:
Masked Siamese Networks for Label-Efficient Learning
https://arxiv.org/abs/2204.07141
Edit: Wang Jing
- END -
Zhoukou colleges and universities have ushered in students to return to school
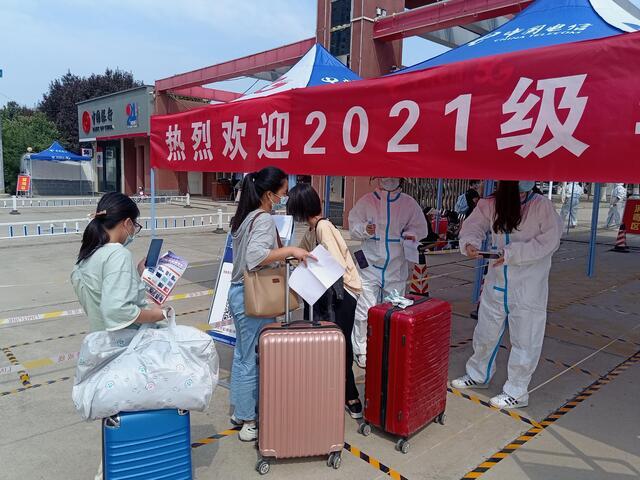
Zhoukou Daily · Zhou Dao client reporter Liu Hua Zhiwen/PictureIt is another year...
Today, Changji people have turned this one!
I do n’t know the moon in hours.It is also suspected of Yao Terrace, flying in the Qingyun.Today is my country's traditional festival Mid -Autumn Festival festivalIt is also the 38th Teacher's Day in...