Chen Xu: Algorithms need to take into account user satisfaction and commodity fairness
Author:Zhongxin Jingwei Time:2022.07.15
Zhongxin Jingwei, July 15th (Wang Yuling) Recently, the "Algorithm Fair Governance and Realization" seminar hosted by the Digital Economy and Legal Innovation Research Center of Foreign Economic and Trade University was held online. Chen Xu, assistant professor of the School of Artificial Intelligence, Renmin University of China, introduced the fairness of the field of recommendation algorithms.
The recommended algorithm is the component of the major websites such as Toutiao, Youku, etc. It can alleviate the problem of information overload, help users save a lot of time, and find the products, videos and other information they are interested in. It can also help companies increase sales. The Internet is widely used.
Chen Xu introduced that from the perspective of artificial intelligence, the recommendation algorithm and traditional artificial intelligence, including computer vision and natural language processing, are different from objective AI, the former is biased to subjective AI, and fairness indicators are more important for it. Due to the differences of users of the recommendation system, based on the user's fairness, in the process of implementation, it pays more attention to the fairness of different groups of users' satisfaction with the satisfaction of the recommendation results, and is not limited to the consistency of the pursuit of recommendation results. The subjective AI is the purpose of improving the benefits of the interest side, not simply improving the accuracy. The interest side includes users, merchants, and other personnel, such as riders. "The range of benefits is much larger than accuracy, and fairness is an important aspect of benefits." Chen Xu said.
Regarding the fairness of the recommended system field, Chen Xu divided it into three categories, including user fairness, commodity -based fairness, and the fairness of users and goods. Based on the fairness of users, there are two commonly used definitions. The first is to be as similar as possible for users in different groups. However, the recommendation results are similar, and the satisfaction of individuals may be reduced, so on this basis, people propose the fairness of user satisfaction. For example, for different users, they do not need to recommend similar results to them, and only need to make different users similar to similar satisfaction. If some users are more satisfied with and some users are relatively low, they are considered unfair. Therefore, the fairness of satisfaction based on the recommendation results is currently highly respected in the field of recommendation system. Based on the fairness of goods hopes that the probability of popular products is slightly lower, and the probability of being recommended by unpopular goods is as high as possible. Based on the fairness of users and products, the above two methods are combined.
From the perspective of implementation, a basic recommendation algorithm based on fairness can usually be divided into three categories. The first is the previous processing method. Such methods often remove sensitive variables or adjust the weight of sensitive variables and predictive results by adjusting weights and deleting samples. Independent independence; the second is the mid -processing method. It hopes that the result of the model learning is fair, without pre -processing the data; the third is the post -processing method. In this method, people usually pre -training the recommended model first , And then sort the recommendation results so that it can meet the fair constraints of groups or individuals. For the fairness of the recommendation algorithm, three issues still need to be studied in the future: first, how can individuals feel whether the algorithm is fair; second, there are many definitions of algorithm fairness. For multi -sensitive variables, especially the contradictory sensitive variables, how to weigh fairness. (For more report clues, please contact Wang Yuling, the author of this article: wangyuling@chinanews.com.cn) (Zhongxin Jingwei APP)
Copyright Copyright Copyright, without written authorization, no unit or individual may reprint, extract or use it in other ways.
Editor in charge: Li Zhongyuan
Pay attention to the official WeChat public account of JWVIEW (JWVIEW) to get more elite financial information.
- END -
IPv6 great navigation, sail points to strong application
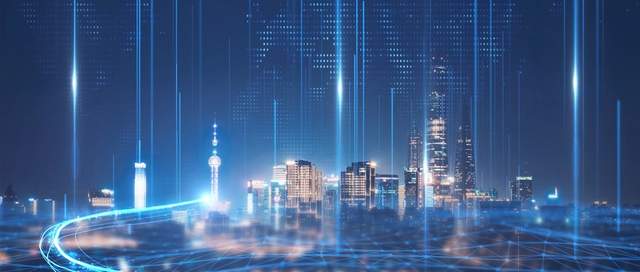
Looking back at the digitalization process for decades, we will find a phenomenon:...
Following the smart home, the robot has also been split, Hikvision will gradually split the high -gr

Text/Yang JianyongFor a long time, Hikvision's operation has maintained a steady g...