This mathematics book AI circle is all turning. The senior ML researcher is 7 years. The free electronic version can be seen
Author:Quantum Time:2022.07.02
发 发 | Public account QBITAI
In the past few days, a free math tutorial has been rotated in the machine learning circle.
This book is called Probabilistic Numerics. The author is three machine learning from Mapo, Oxford and Inria, and one of the Google academic references reached 17000+.
The three authors of Philipp Hennig, Michael A. Osborne and Hans P. Kesting wrote this book for a total of 7 years, more than 400 pages in length.
After the release of the new book, one of the author Philipp Hennig sighed on Twitter: it finally came out.
In addition to the entities, the author also shared the free electronic version of the book on the Internet. (PS: You can view it at the end of the text ~)
Specifically, this book hopes to teach everyone how to optimize machine learning models from the perspective of mathematics.
Generally, machine learning involves a large number of linear algebra, points, or the minimum value of finding non -linear functions, and these problems often occupy a large amount of computing resources.
Therefore, the author hopes to explain the principle of calculation of the probability value behind, let us understand the "reasons for energy consumption" of the model, so as to better optimize the model.
For this book, Antonio Vergari, a machine learning lecturer at the University of Edinburgh, gave a praise:
Numerous calculation points are not only the core of machine learning, artificial intelligence, but also the core of engineering and physical fields. In addition to the perspective of numerical analysis, from the perspective of model reasoning and dissemination uncertainty, it is also very valuable.
What is the use of this book?
In the computer, there are many problems involving the solution of complex systems.
For example, the use of computers to predict weather and cancer cell gene mutations, which are related to complex systems. Because this system is easy to introduce uncertainty when solving, it will also cause waste of computing resources.
In machine learning, the prediction results given by the model are not necessarily reliable, and various factors will also cause uncertainty of the output result.
At present, with the improvement of data accuracy, the amount of calculation caused by uncertainty is still increasing.
From a mathematical perspective, how to quantify this uncertainty and reduce the waste of computing resources, it involves the theoretical and methods of probability values (probability statistics, numerical analysis, etc.).
If you can master its theoretical skills, computers can process data more efficiently, and can also use this uncertainty to make the best decision -making, including the use of Bayesian inference theory to build more flexible and more efficient efficiency , More personalized algorithm.
In the book, the author not only fully explains the principle of probability value calculation, but also further teaches you how to optimize a machine learning model through practice questions, and all important exercises are equipped with solutions.
This was praised by netizens.
As the author said in the profile:
We wrote this book for all those who need to use numerical calculations, whether it is a celestial physicist or a deep learning hacker. For those who are being or intended to be developers in the field of value calculation, and those who are learning machine learning, we hope that this book will be interesting.
What did this book say?
At the moment of the rapid development of machine learning, this book aims to make an overview of the emerging probability of value.
The content of the book is mainly from the following aspects:
1. Mathematical basis
Probability Calculation is a bridge between machine learning and application mathematics. To learn machine learning, mathematics is a topic that cannot be around.
This chapter introduces key concepts such as probability reasoning, Gaussian functions, regression, and linear algebra that will be used in the future.
Readers with statistical or machine learning background will be easy to read ~
2. Points
This chapter uses the basic concept of points to introduce the core of the probability value calculation -the theory of Bayesian accumulation, the classic accumulation formula, etc., and reconstruct the existing numerical values. Essence
3. Linear algebra
Linear algebraic operations can be said to be the most basic numerical calculation. Like matrix operations and vector operations, they are almost the cornerstone of all heavyweight operations in the contemporary computer. Therefore, research in this field is very deep.
First of all, you need to understand the basic knowledge of some linear algebra -vectorization matrix, Cronek accumulation, Zhengding matrix, Frobenius matrix numeric number, and so on.
Secondly, the book is committed to cultivating intuitive understanding of linear algebra, which helps us better understand the nature of mathematics and understand the simplest routine behind complex formulas.
4. Local optimization
In the process of numerical calculations, if improper modeling will cause many problems, this is particularly obvious in the fields of deep learning.
This chapter focuses on the issue of non -linear optimization, and explains as an example in step -length selection.
5. Global optimization
This chapter introduces a very effective global optimization algorithm -Bayeste Optimization. This method can be unlimited to solve the problem of high calculation costs and unknown guidance, and can find the global minimum value in as few steps as possible.
6. Frequent differential equations solve methods
In the process of studying the constant differential equation, we need to dialectically look at the relationship between frequent differential equations and partial differential equations, and we need to transform it in a timely manner. In this way, we can flexibly solve the constant differential equation.
This chapter is mainly explained from the classic ODE solution, ODE filter peace skids, and other aspects. 7. Prospect
The future of probability values is vast, and many basic mathematics, engineering and philosophical issues in this field still need to be resolved. In this chapter, we will focus on discussing some open issues that may affect the development of the academic world at least in the next ten years.
8. Practice & Answer
In addition to the above text materials, this book also provides many practice questions with solutions.
Who are the three authors?
One of the author's Michael A. Osborne shared on Twitter, "We started writing this new book on machine learning from 2015, now it grows like my child."
Michael A. Osborne, a professor of machine learning at Oxford University, is also the co -founder of Mind Foundry Co., Ltd.
OSBORNE focuses on the direction of active learning in the field of machine learning, Bayeste Optimization, and Bayesian points, and is keen on the emerging probability value field.
Philipp Hennig, a professor of machine learning methods of Tibergen University, is also a part -time researcher at MPII (Max Planck Intelligent System Research Institute), Ellis (European Learning and Smart System Laboratory) learning machine theory, algorithm and computing research project Joint Director.
In his career, the probability numerical method is one of the main research directions. Hennig's research was supported by Emmy Noether, Max Planck, and ERC Scholarship.
Hans P. Kesting, post -doctoral researcher of Inria (Institute of French National Information and Automation) and ENS (Paris High Normal University), engaged in machine learning, mainly research directions is Bayesian reasoning, dynamic system optimization, etc.
Finally, attach the "Prosperity value" provided by the author for free electronic version. Interested friends hurry up and see it!
"Probability": https://www.prbabilistic-numerics.org/Assets/probabilisticNumerics.pdf
- END -
Digital transformation, the key is "people", the hardest is "people"
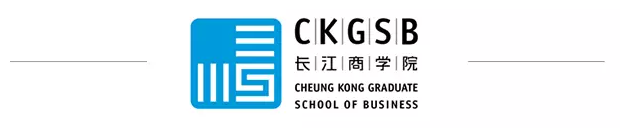
The following article comes from the Economic Observer, the author Liu JinIn the f...
The latest research comes!History of panda can be traced back to 6 million years ago
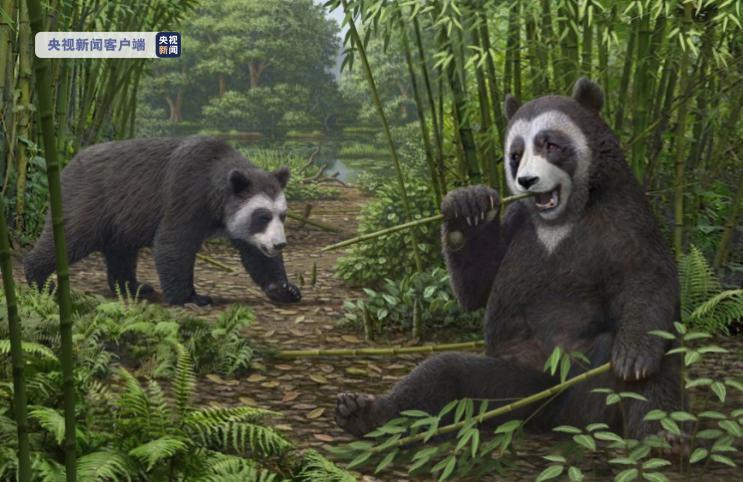
The giant panda belongs to the giant panda is different from its same brothers. It...