How to imitate some parts of the brain
Author:Data School Thu Time:2022.09.29
Source: scienceai
This article is about 2500 words, it is recommended to read for 7 minutes
This article will demonstrate how to improve the performance of the model through threshold tuning.
Understanding how the brain organizes and access space information, what are we in the corner and how to reach there, this is still a difficult challenge. This process involves the entire memory network and storage space data from tens of billions of neurons, and each neuron is connected to thousands of other neurons.
Neurologists have identified key elements, such as neurons in grid cells and mapping positions. But more deeper will be proven to be tricky: not to say that researchers can remove or study human gray slices to observe how the position, sound, and smell memory of positions is flowing and connecting each other.
Artificial intelligence provides another way. Over the years, neurosciences have used a variety of neural networks -engines that provide motivation for most deep learning applications -to simulate the discharge of neurons in the brain.
In recent work, researchers have shown that hippocampus is a brain structure that is vital to memory, and is basically a special neural network, known as Transformer. Their new model tracks space information in a similar way to the brain. They have seen extraordinary success.
We know that these brain models are equivalent to Transformer, which means that our model is better and easier to train. Said James Whittington, a cognitive neurologist from Stanford University.
The research by Whittington and others shows that Transformer can greatly improve the various computing capabilities performed by neural network model simulation mesh cells and other parts of the brain. Whittington said that such a model can promote our understanding of artificial neural networks, and it is even more likely to promote our understanding of how to calculate the brain.
We are not to rebuild the brain. David Ha, a computer scientist at Google brain, said he is also studying the Transformer model, but can we create a mechanism to complete what the brain does?
TRANSFORMERS first appeared five years ago and is a new way for artificial intelligence to deal with language. They are secret weapons in the striking programs such as BERT and GPT-3, which can generate convincing lyrics, create Shakespeare's fourteen lines of poems, and imitate customer service representatives.
Transformers uses a mechanism called self -attention, each input -a word, a pixel, a number in a sequence -always connects to each other input. (Other neural networks only connect to some other inputs.) However, although the converter was designed for language tasks, they later performed well on other tasks, such as classification of images -now are brain model modeling. Essence
In 2020, a group led by computer scientist Sep Hochreiter, a computer scientist at the University of Lindz, Austria, used Transformer to transform a powerful, long -term memory retrieval model, called Hopfield network. Forty years ago, the Princeton physicist John Hopfield first proposed that these networks follow the general rules: at the same time, the active neurons have established a firm connection with each other.
Hochreit and his collaborators pointed out that researchers have been looking for better memory retrieval models, and they see how the Hopfield network retrieves the connection between memory and converter how to perform attention. They upgraded the Hopfield network and basically turned it into a Transformer. Whittington said that due to more effective connection, this change allows the model to store and retrieve more memory. Hopfield himself, together with the Dmitry Krotov in the Mit-IBM Watson AI laboratory, proves that the Hopfield network based on Transformer is reasonable in biology.
Then, earlier this year, Whittington and Behrens helped to further adjust the Hochreiter's method and modify the converter so that the memory can no longer be regarded as a linear sequence -just like a string of words in a sentence -but encoded them into them as a word -but encod Coordinates in high -dimensional space. As researchers say, this twist further improves the performance of the model on neuroscience. They also show that the model is mathematically equivalent to the grid cell discharge mode model that neurosciences see in FMRI scanning.
Caswell Barry, a neuroscience at the University of London, said: Grid cells have this exciting, beautiful, and regular structure, and it is unlikely to be randomly attractive. This new job shows how transformer has accurately copied the models observed in the hippocampus. They realize that Transformer can determine its position based on the previous state and its movement, and combine a way with the traditional grid unit model. Recent studies have shown that Transformers can also promote our understanding of other brain function. Last year, Martin Schrimpf, a computing neuroscience at Massachusetts Institute of Technology, analyzed 43 different neural network models to see how the predictive effects of the measurement results of human neural activity reports on FMRI and cortex electrocardiogram. He found that Transformers is currently the leading and most advanced neural network, which can predict almost all changes found in imaging.
HA and computer scientist Yujin Tang recently designed a model. This model can deliberately send a large amount of data through random and disorderly way through Transformer to imitate how the human body observes the sensory observation to the brain. Their Transformer, like our brain, can successfully process disorderly information flow.
The neural network is inherently accepting specific inputs. Tang said. But in real life, data sets often change very quickly, and most AI cannot adjust. We want to test a architecture that can quickly adapt.
Despite these signs of progress, Behrens believes that Transformers is only a step towards an accurate brain model, not the end of the exploration. I must be a suspicious neuroscience here. He said, for example, I don't think Transformers will eventually become the way we think about language in the brain, even if they have the best sentence model currently.
Is this the most effective basis for predicting where and what will I see next? Honestly, it is too early to say. Barry said.
Schrimpf also pointed out that even the best -performing converter is limited. For example, it performs well in terms of words and phrases, but it is not applicable in larger language tasks such as storytelling.
My feeling is that this architecture, this transformer, allows you to enter the correct space to understand the structure of the brain, and can be improved by training. Schrimpf said that this is a good direction, but this field is super complicated.
Related reports: https://www.quantamagazine.org/how-ai-transFormers-mimic-parts-of-the-brain-20220912//20220912/
Edit: Huang Jiyan
- END -
Countdown!100 million yuan consumer coupon, remember to receive →
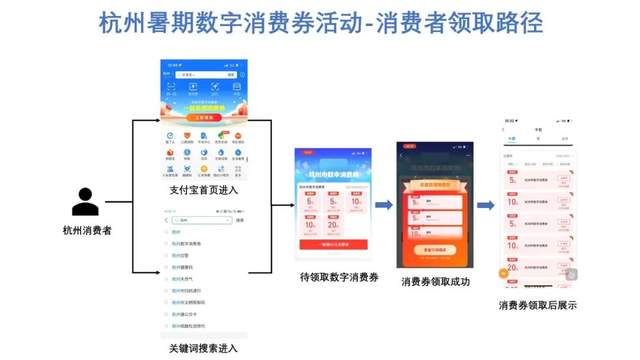
Hangzhou will issue the first summer digital consumer coupon for the first issue o...
EU: By 2024, the Type-C will be forced as a mobile phone charging port
Comprehensive Reuters and Germany News Agency, on June 7, local time, the negotiating representatives of the European Parliament and 27 EU member states agreed that mobile phones and other electroni