[Harducleic Book] Machine learning confrontation of robustness
Author:Data School Thu Time:2022.09.21
Source: Specialty
This article is introduced by books. It is recommended to read for 5 minutes
This book introduces common algorithms for confrontation attacks, defense and verification.
The confrontation of machine learning describes the latest progress of the topic and introduces common algorithms for confrontation attacks, defense and verification. The chapter covers confrontation attacks, verification and defense. It mainly focuses on image classification applications. This is the standard benchmark for countermeasures to ride. Other parts discussed anti -examples other than image classification, other threat models other than testing time, and applications against robustness. For researchers, this book provides a comprehensive literature review, summarizing the latest progress in this field, and can be used as a good reference for future research. In addition, this book can also be used as a textbook for graduate courses to teach confrontation or reliable machine learning. Although machine learning (ML) algorithms have achieved significant performance in many applications, recent studies have shown that they lack robustness in confrontation disturbances. The lack of robustness brings security problems to the ML model of practical applications (such as autonomous vehicles, robot control and medical care systems).
https://www.elsevier.com/Books/adversarial-robustness-Machine- Learning/978-0-12-824020-5
With the latest progress of machine learning theory and algorithms, the design of high -capacity and scalable models, such as neural networks, rich data sets and sufficient computing resources, machine learning (ML), or more widely, artificial intelligence (AI ), Have changed our industry and society at an unprecedented speed. When we look forward to the positive impact of machine learning technology, we often ignore the potential negative effects, which may bring considerable moral concerns, and even cause setbacks due to laws and regulations and disastrous failures, especially For key tasks and high -risk decision -making tasks. Therefore, in addition to accuracy, trustworthy machine learning is the last milestone based on machine learning technology realization and development. Trusted machine learning contains a series of basic themes, such as countermeasures, fairness, explanatoryness, accountability and ethics.
The focus of this book is to evaluate, improve and use the efforts to evaluate, improve, and use robustness to achieve better and more trusted versions. Using unwilling machine learning as a vulnerability, creating an unattended entrance for the intentional party, manipulating machine predictions, and avoiding human attention at the same time to obtain your own interests. No matter what the role of a person in ML, as a model developer, stakeholder, or user, we believe that everyone must understand the confrontation of machine learning, just like understanding the performance and restrictions of their vehicles before driving. Essence For model developers, we advocate active internal robust tests on your own models and systems to perform errors and reduce risks. For stakeholders, we advocate acknowledging the weaknesses that may exist in products and services, and in a forward -looking manner to conduct honest and thorough risk and threat assessment to prevent income/reputation loss and catastrophic damage to society and the environment. For users who use machine learning by -products, we advocate actively understand the limitations of their safe use and understand possible misuse. These aspects related to robbing, as well as available technologies and tools, explained in this book.
Generally speaking, the study of the worst situation in machine learning in machine learning, while standard machine learning practice pays attention to the average performance, such as predicting the accuracy of test data sets. The concept of analysis of the worst situation is stimulated by ensuring that the machine learning is stimulated by the changes in the training environment and deployment scenarios and the necessity of accurate prediction. Specifically, this change may be caused by natural events (for example, data drift caused by different light conditions) or malicious attempts (for example, a hacker aimed at compromising and obtaining machine learning system/service control) Essence Therefore, instead of asking "How does machine learning on a given data set/task?" In the confrontation, we asked, "If the data set or model can go through different quantitative levels, machine learning What is the robustness and accuracy? "This intervention process usually involves the introduction of virtual opponents in machine learning for robust assessment and improvement, which is a key factor in confrontation machine learning.
This book aims to provide a overall overview of confrontation, covering the life cycle of machine learning, from data collection, model development, to system integration and deployment. Content provides a set of comprehensive research technology and practical tools for machine learning confrontation. This book covers the following four research focus on confrontation: (i) attack-finding the failure mode of machine learning; (ii) defense-strengthening and protecting machine learning; certification-formulating proven stable performance guarantee guarantee He (IV) Application -based on confrontation robust research and invention new use cases.
We summarize the content of each part of this book as follows.In part 1, we introduced the preliminary content of this book, linked conflict robustness with confrontation machine learning, and provided interesting discoveries to motivate confrontation.In part 2, we introduced different types of confrontation attacks to make different assumptions on the attackers' knowledge of the life cycle of machine learning, the realization of the target machine learning system knowledge, digital and physical space, and the capabilities in the data mode.In part 3, we have introduced quantitative neural networks to prove a rigid level certification technology.In part 4, we will introduce the robust defense of machine learning against confrontation attacks.Finally, in part 5, we introduced several new applications inspired by machine learning confrontation research.
- END -
Story about the original black hole
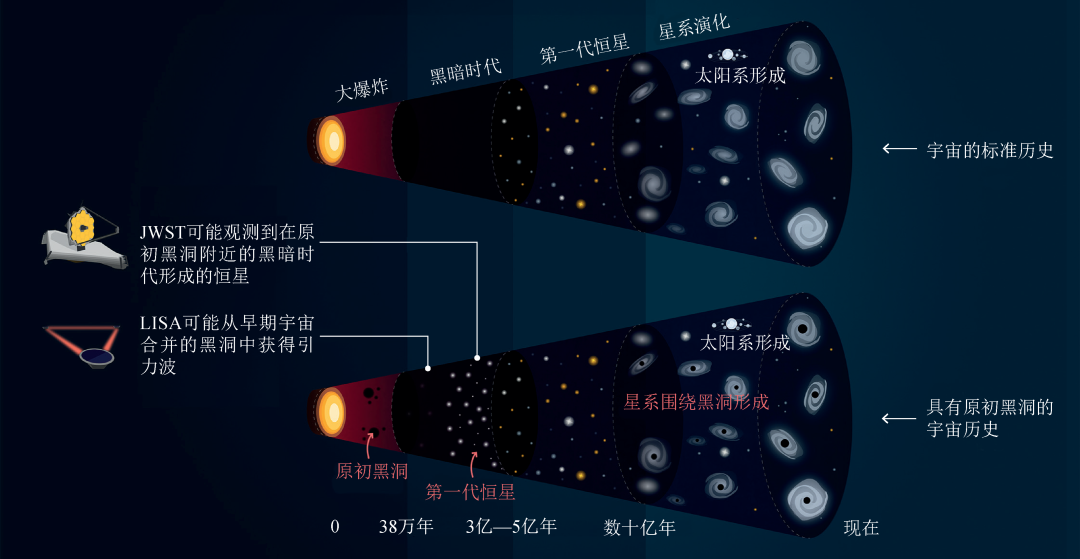
Deng Shuxia and Gou Lijun compiled from Ian Randall. Physics World, 2022, (5): 33T...
Technical defense+civil defense!State Grid Yongjia County Power Supply Company fully faced the typhoon "Plum Blossom"
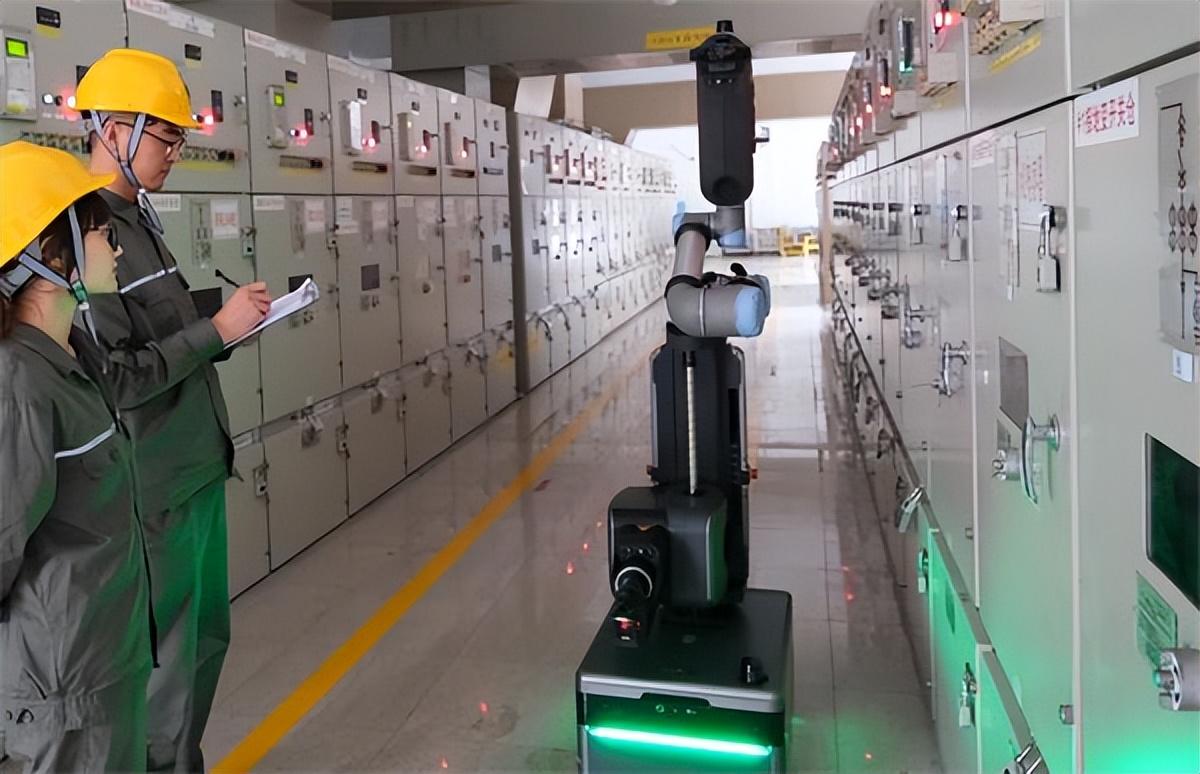
At 9 am on September 14th, the impact of Typhoon Plum Blossom on Yongjia County on...