The more AI evolves, the more like the human brain!Meta found the machine's "frontal leaf cortex" hot discussion
Author:Quantum Time:2022.06.20
Fish and sheep Xiao Xiao is from the quantity of the Temple of Temple | Public Account QBITAI
You may not believe it. One AI has just been proven that the way of dealing with the voice is similar to the mystery of the brain.
Even the structure can correspond to each other-
Scientists directly positioned "visual cortex" on AI.
Once the research from Meta AI and other institutions was out, it immediately exploded on social media. A large wave of neurosciences and AI researchers went to watch.
Lecun praises this is "excellent work": self -monitoring Transformer layered activities are really closely related to human auditory leather activities.
Some netizens took the opportunity to tease: Sorry Marcus, but AGI is really coming.
However, research has also caused some scholars' curiosity.
For example, Patrick MineAult, a doctor of neuroscience at McGill University, asked:
We published in a paper in Neurips and tried to connect FMRI data with the model, but at the time I didn't think these two had something to do.
So, what kind of research is this, and how does it come to the conclusion that "this AI is working like a brain"?
AI learns to work like a human brain
Simply put, in this study, researchers focused on the problem of voice processing and compared the self -supervision model WAV2VEC 2.0 with 412 volunteers' brain activities.
Of the 412 volunteers, 351 people spoke English, 28 people spoke French, and 33 people spoke Chinese. The researchers listened to them for about an hour, and in this process, they used FMRI to record their brain activities.
On the model, the researchers use the labelless voice for more than 600 hours to train WAV2VEC 2.0.
Corresponding to the mother tongue of volunteers, the model is also divided into three models: English, French, and Chinese, and another one is trained in data sets of non -phonetic acoustic scenarios.
Then these models also listened to the same audio book. Researchers extracted the model activation.
Related evaluation standards, follow this formula:
Among them, X is the model activation, Y is the human brain activity, W is the standard coding model.
From the results, self -supervision and learning really allows WAV2VEC 2.0 to produce a brain -like voice representation.
As can be seen from the above figure, in the primary and secondary auditory cortex, AI obviously predicts the brain activity of almost all cortex areas.
Researchers have further discovered which layer of AI's "hearing cortex" and "frontal leaf cortex".
The figure shows that the hearing cortex is the most consistent with the first layer (blue) of Transformer, while the frontal cortex coats with the deepest layer (red) of Transformer.
In addition, researchers quantify the ability differences between human perception of mother tongue and non -mother phonetic, and compare with the WAV2VEC 2.0 model.
They found that AI also has a stronger identification ability like "mother tongue". For example, French models are easier to perceive the stimulus from French than English models.
The above results prove that the 600 -hour self -supervision learning is enough to allow WAV2VEC 2.0 to learn the specific characteristics of the language -this is equivalent to the "data volume" in the process of learning to speak.
You know, before the DeepSpeeeeeech2 paper believes that it takes at least 10,000 hours of voice data (the one that is marked) in order to build a set of good voice transfer text (STT) system.
Reconnect neuroscience and AI discussion again
For this study, some scholars believe that it has indeed made some new breakthroughs.
For example, Jesse Engel from Google's brain said that the study raised visualized filters to a new level.
Now, not only can they see what they look like in "pixel space", but even their appearance in "brain -like space" can be simulated:
For example, former Mila and Google researcher Joseph Viviano believes that this study also proves that the Resting-State imaging data in FMRI is meaningful.
But in a discussion, there were also some doubts.
For example, Patrick MineAult, a doctor of neuroscience, not only pointed out that he had done similar research, but did not conclude that he also gave some questions.
He believes that this study does not really prove that it measures the process of "voice processing".
Compared to the speed of people talking, the speed of the FMRI measurement signal is actually very slow, so the conclusion that "WAV2VEC 2.0 learns the brain" is unscientific.
Of course, Patrick Mineault said that he was not denying the research point. He was also "one of the author's fans", but this research should give some more convincing data.
In addition, some netizens believe that the input of WAV2VEC and the human brain is also different. One is the waveform after treatment, but the other is the original waveform.
In this regard, one of the author, Meta AI researcher Jean-Rémi King summarizes:
The intelligence of human level is indeed a long way to go.But at least now, we may be on a correct road.what you think?
- END -
Huawei released two major ascension artificial intelligence plans to promote the development and scientific research innovation of AI talents
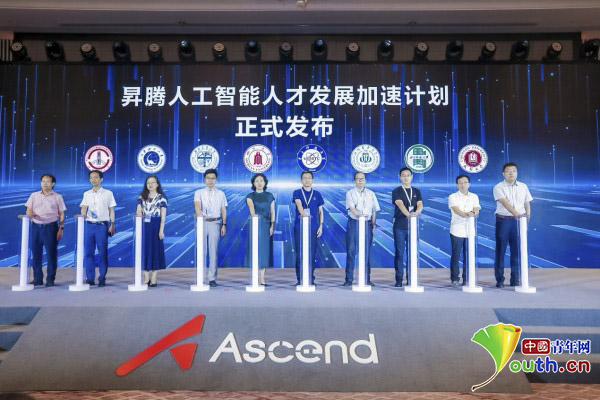
China Youth Net, Chengdu, June 25 (Reporter Li Yongpeng) On the Chengdu Station of...
Qingdao Industrial Internet "Four New Leadership" Western Enterprise Monthly launched

In order to promote the construction of Gongfu Qingdao • Intelligent Manufacturin...