Multi -mode image synthesis and editor are so hot, Mapu Institute and Nanyang Polytechnic have made a detailed review
Author:Data School Thu Time:2022.08.29
Source: Heart of the machine
This article is about 1,000 words, it is recommended to read for 5 minutes
In this summary summary of the existing multi -mode image synthesis and editing methods, the current challenges and future directions of the field are discussed and analyzed.
Recently, the Dalle-2 and Google released by OpenAI have achieved amazing text to the generating effect of images, which has caused widespread attention and derived a lot of interesting applications. The generation from text to images is a typical task in the field of multimodal image synthesis and editing.
Recently, researchers from Mapo and Nanyang Polytechnic and other institutions have investigated and analyzed the current status and future development of multi -mode image synthesis and editing.
Thesis address: https: //arxiv.org/pdf/2112.13592.pdf
Project address: https://github.com/fnzhan/mise
In the first chapter, the review describes the significance and overall development of multi -mode image synthesis and editing tasks, as well as the contribution and overall structure of this paper.
In the second chapter, according to the data modal of the guidance picture synthesis and editing, the schedule introduces more commonly used visual guidance (such as semantic diagrams, key points, edge diagrams), text guidance, voice guidance, scene diagram (Scene Graph) guidance and corresponding modular data processing methods and unified representation frameworks.
In the third chapter, according to the model framework of image synthesis and editing, the paper classified the current methods, including GAN -based methods, self -regression methods, diffusion model methods, and neuroma radiation fields (NERF) methods.
Because GAN -based methods generally use conditions GAN and unconditional GAN, this paper further divides this category into modal conditions (such as semantic diagrams, edge diagrams), cross -modal conditions (such GAN's anti -discrimination (unified modal) was described in detail.
Compared with GAN -based methods, the self -regression model method can handle multi -mode data more naturally and use the currently popular Transformer model. The self -regression method generally learn a vertical quantitative encoder to represent the picture discrete tract as the token sequence, and then the distribution of the token distribution from the regression type. Because text and voice can be expressed as a token and as a condition for self -regression modeling, various multi -mode picture synthesis and editing tasks can be unified into a framework.
Recently, the fiery diffusion model has also been widely used in multi -mode synthesis and editing tasks. For example, the amazing Dalle-2 and Imagen are implemented based on the diffusion model. Compared with GAN, the diffuse -generating model has some good properties, such as static training goals and easy scalability. The paper classified and detailed analysis of the existing methods based on the condition diffusion model and the pre -training diffusion model.
The above methods are mainly focused on multi -mode synthesis and editing of 2D images. With the rapid development of the neuroma field (NERF), 3D perception multi -mode synthesis and editors have also attracted more and more attention. Due to the need to consider multi -perspective consistency, 3D perception multi -mode synthesis and editors are more challenging tasks. This article is aimed at the three methods of NERF for a single scenario to optimize NERF, and the three methods of generating NERF and NERF classified the existing work.
Subsequently, the review compared and discussed the above four model methods. Overall, compared to GAN, the most advanced model currently prefers the self -regression model and diffusion model. The application of NERF in multi -mode synthesis and editing tasks opened a new window for research in this field.
In the fourth chapter, the review brings together data sets popular in the field of multi -mode synthesis and editors and corresponding model labeling, and for typical tasks of various modes (semantic image synthesis, text to image synthesis, voice guidance image editing)) A quantitative comparison of the current method.
In the fifth chapter, this review has discussed and analyzed the current challenges and future directions of this field, including large -scale multimodal data sets, accurate and reliable evaluation indicators, efficient network structures, and 3D perception development direction Essence
In Chapter 6 and 7, the summary of the potential social influence and summary of the content and contribution of the article respectively explained the content and contribution of the article.
Edit: Huang Jiyan
- END -
The new type of environmental protection air conditioner came out!Hydrinating hydrocarbons that instead of strong temperate effect
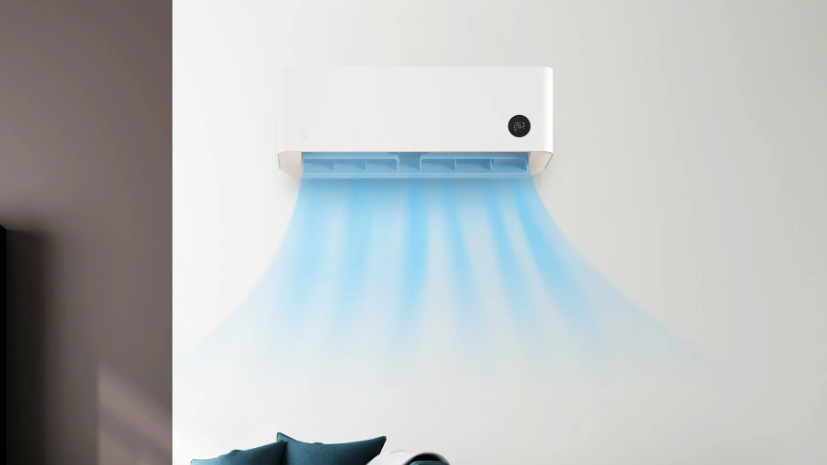
Researchers reported a prototype equipment that can replace the existing air condi...
The commended test questions are getting harder and harder
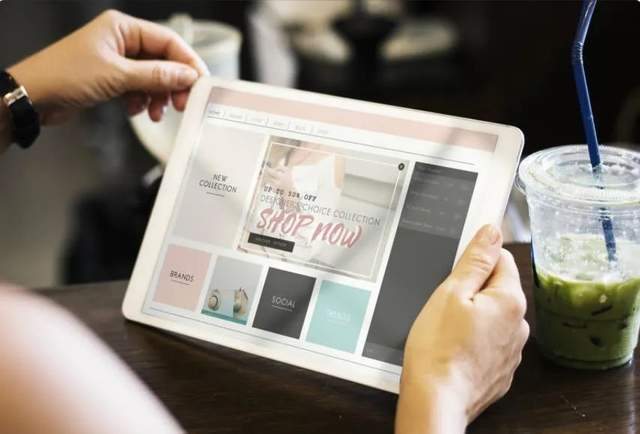
The picture comes from Canva.In the past few years, traffic anxiety on Internet la...